Evaluation of YOLOv7 Deployment Performance on Various Platforms (CPUs, GPUs) and Strategies
DOI:
https://doi.org/10.14464/ess.v10i8.660Abstract
In the past few years, machine learning (ML) has evolved from academic research curiosity into a practical domain capable of addressing tangible business challenges.
Deploying machine learning (ML) models to production with an equivalent degree of thoroughness and automation as conventional software systems has proven to be a complex endeavor, demanding additional attention and infrastructure to address the unique challenges involved.
Building and training machine learning models is just one part of the process. Deploying models into production is a critical step that brings the potential of these models to life and allows them to deliver value to organizations and end users.
It's the bridge between the theoretical work in the research and the practical application of machine learning in real-world scenarios. This research paper presents an original study focused on deploying a traffic sign detection and recognition system utilizing the YOLOv7 algorithm. The study evaluates the algorithm's performance across diverse platforms and determines a robust production deployment strategy for YOLOv7.
Index Terms—Traffic sign, Detection, Classification, Deep Neural networks, YOLOv7, Deployment
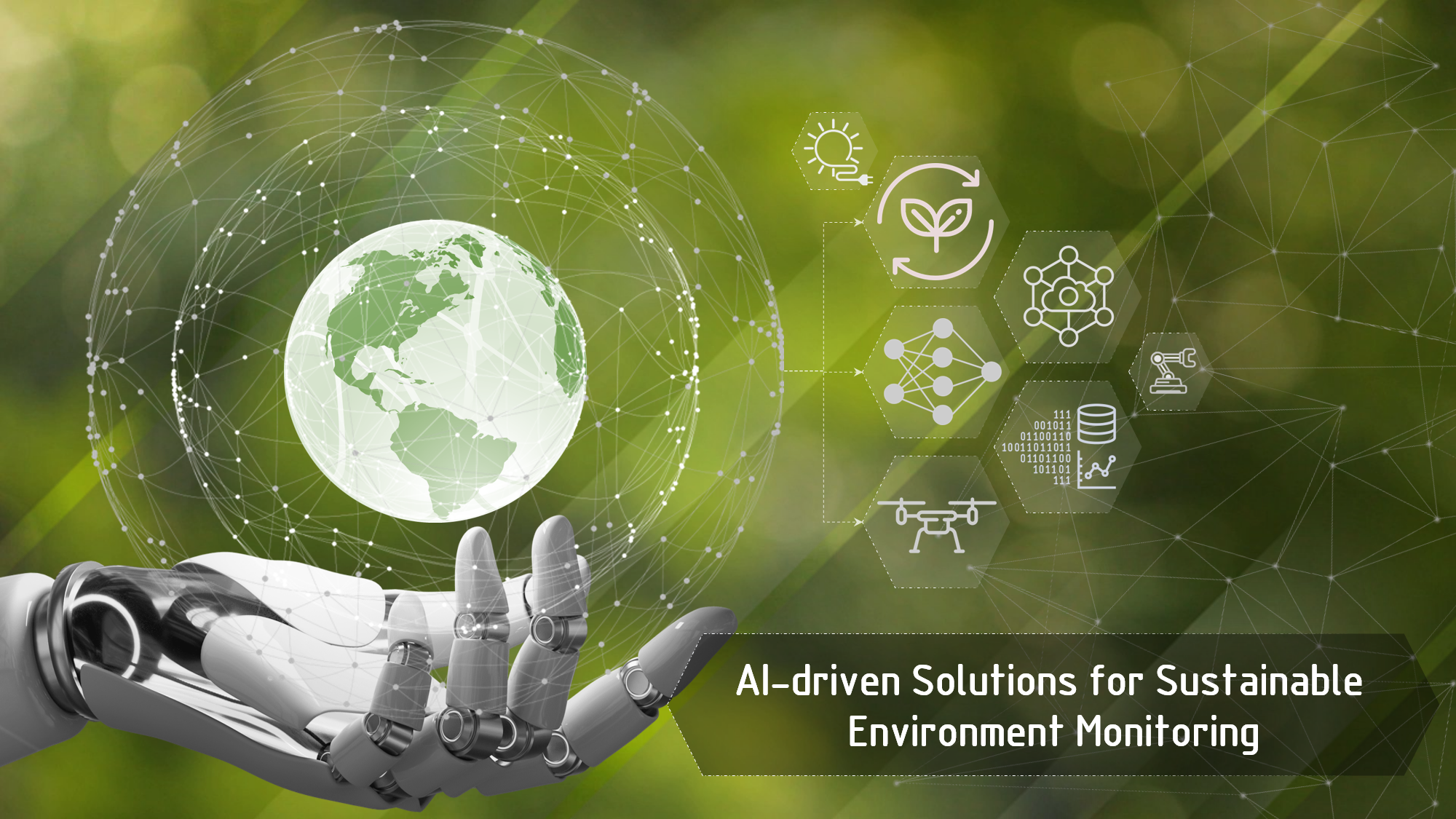
Downloads
Published
Issue
Section
License
Copyright (c) 2023 Fatima Abouzid, Shadi Saleh, Salim Harras Harras, Wolfram Hardt, Ghennioui Hicham

This work is licensed under a Creative Commons Attribution 4.0 International License.
Copyright for articles published in this journal is retained by the authors. The content is published under a Creative Commons Licence Attribution 4.0 International (CC BY 4.0). This permits use, distribution, and reproduction in any medium, provided the original work is properly cited, and is otherwise in compliance with the licence.