Automated Identification of Wood Surface Defects Based on Deep Learning
DOI:
https://doi.org/10.14464/ess.v10i7.627Abstract
Wood plates are widely used in the interior design of houses primarily for their aesthetic value. However, considering its esthetical values, surface defect detection is necessary. The development of computer vision and CNN-based object detection methods has opened the way for wood surface defect detection process automation. This paper investigates deep-learning applications for automatic wood surface defect detection. It includes the evaluation of deep learning algorithms, including data generation and labeling, preprocessing, model training, and evaluation. Many adjustments regarding the dataset size, the model, and the modification of the neural network were made to evaluate the model's performance in the specified challenge. The results indicate that modifications can increase the YOLOv5s performance in detection. The model with GCNet added and trained in 4800 images has achieved 88.1% of mAP. The paper also evaluates the time performance of models based on different GPU units. The results show that in A100 40GB GPU, the maximum time to process a wood plate is 2.2 seconds. Finally, an Active learning approach for the continual increase in performance while detecting with the smaller size of manual labeling has been implemented. After detecting 500 images in 5 cycles, the model achieved 98.8% of mAP. This scientific paper concludes that YOLOv5s modified model is suitable for wood surface defect detection. It can perform with high accuracy in real time. Moreover, applying the active learning approach can facilitate the labeling process by increasing the performance during detection.
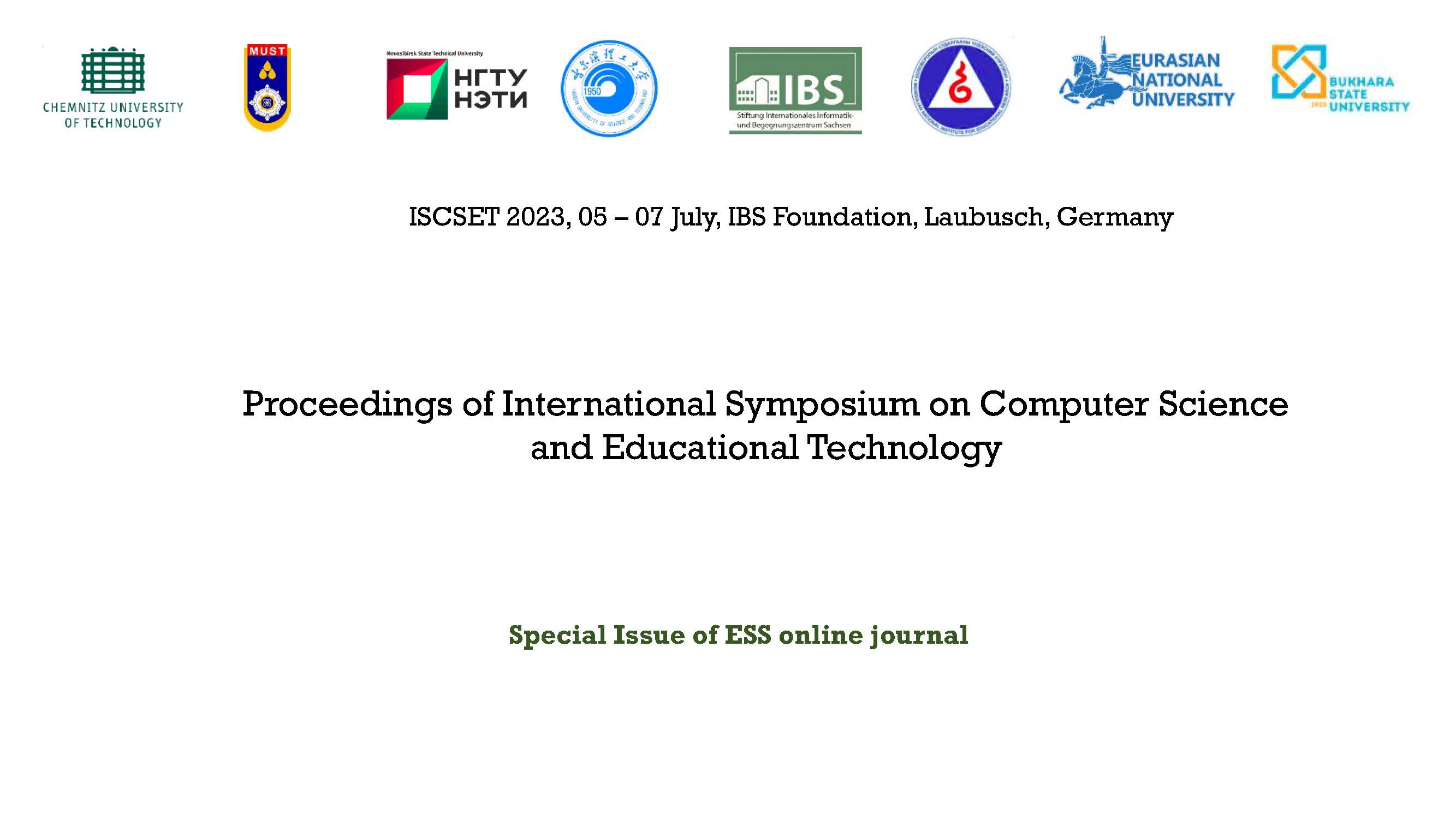
Downloads
Published
Issue
Section
License
Copyright (c) 2023 Besfort Syla, Shadi Saleh, Prof. Dr. Dr. h. c. Wolfram Hardt

This work is licensed under a Creative Commons Attribution 4.0 International License.
Copyright for articles published in this journal is retained by the authors. The content is published under a Creative Commons Licence Attribution 4.0 International (CC BY 4.0). This permits use, distribution, and reproduction in any medium, provided the original work is properly cited, and is otherwise in compliance with the licence.