Exploring SSD Detector for Power Line Insulator Detection on Edge Platform
DOI:
https://doi.org/10.14464/ess.v10i5.603Abstract
Power line insulator detection is pivotal for the consistent performance of the entire power system. It forms the basis of Unmanned Aerial Vehicle (UAV) inspection, an emerging trend in power line surveillance. This paper addresses the challenge of insulator detection in cluttered aerial images, given the constraints of a limited dataset and lower computational resources, specifically on the NVIDIA Jetson Nano platform. We have developed two approaches based on active and passive deep learning algorithms, underpinned by the Single Shot Multibox Detector (SSD) meta-architecture with MobileNetV2 as its backbone - SSD300 and SSD640. The proposal models managed a frame rate of 9 fps in 10W power mode and 5.6 fps in 5W power mode. Our experiments demonstrated that the proposed active learning model could conduct robust insulator detection, achieving a mAP of 94.5% while using only 43% of the total dataset, comparable to the traditional deep learning approach's 94.6% mAP using the entire dataset. Significantly, the active learning model seeks feedback during the training process, enabling it to learn from its mistakes and enhance accuracy over time. This also contributes to improved generalizability and interpretability of the model by seeking diverse and representative samples during training, all while reducing the computational and annotation overhead.
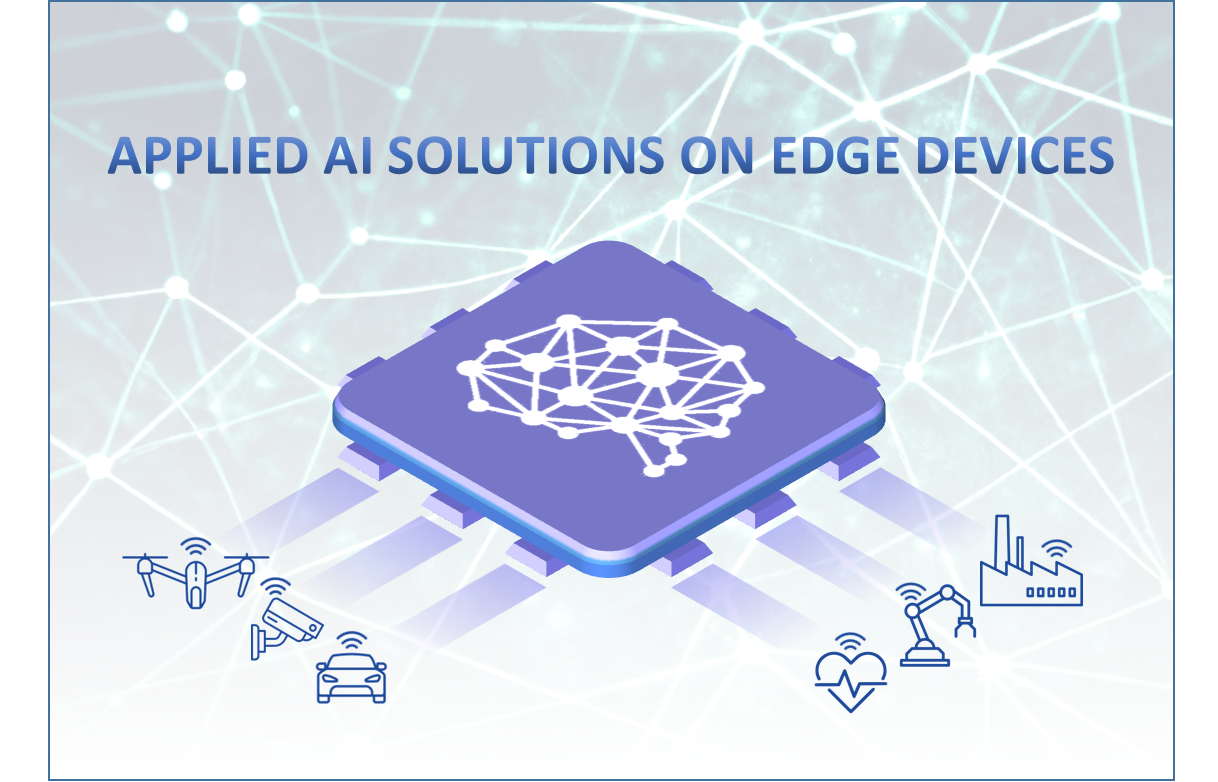
Downloads
Published
Issue
Section
License
Copyright (c) 2023 Mohamed Salim Harras, Soaibuzzaman, Shadi Saleh, Wolfram Hardt

This work is licensed under a Creative Commons Attribution 4.0 International License.
Copyright for articles published in this journal is retained by the authors. The content is published under a Creative Commons Licence Attribution 4.0 International (CC BY 4.0). This permits use, distribution, and reproduction in any medium, provided the original work is properly cited, and is otherwise in compliance with the licence.