Fruit Classification Based on Improved YOLOv7 Algorithm
DOI:
https://doi.org/10.14464/ess.v10i7.600Abstract
With the rapid development of technology and advancements, unmanned vending machines have emerged as the primary contactless retail method. The efficient and accurate implementation of automated identification technology for agricultural products in their distribution and sales has become an urgent problem that needs to be addressed. This article presents an improved YOLOv7 (You Only Look Once) algorithm for fruit detection in complex environments. By replacing the 3×3 convolutions in the backbone of YOLOv7 with Deformable ConvNet v2(DCNv2), the recognition accuracy and efficiency of fruit classification in YOLOv7 are significantly enhanced. The results indicate that the overall recognition accuracy of this system for ten types of fruits is 98.3%, showcasing its high precision and stability.
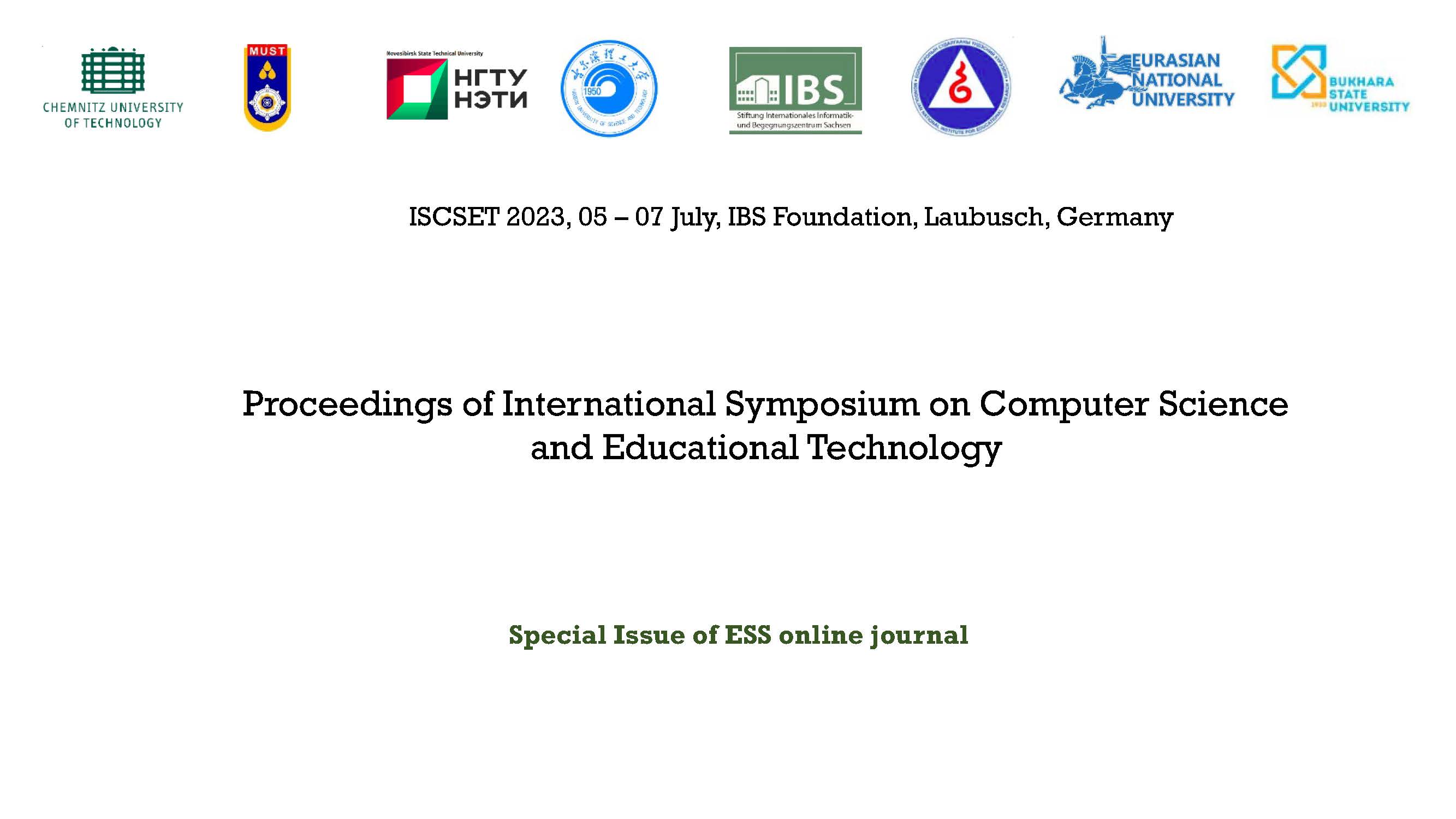
Downloads
Published
Issue
Section
License
Copyright (c) 2023 Shibo Guo, Tianyu Ren, Qing Wu, Xiaoyu Yu, Aili Wang

This work is licensed under a Creative Commons Attribution 4.0 International License.
Copyright for articles published in this journal is retained by the authors. The content is published under a Creative Commons Licence Attribution 4.0 International (CC BY 4.0). This permits use, distribution, and reproduction in any medium, provided the original work is properly cited, and is otherwise in compliance with the licence.